Over the past three years, the Saturday Morning NeuroSim group has met weekly on Saturdays (mornings in North America). The Saturday Morning format continues in the tradition of Saturday Morning Physics and covers a wide variety of topics.
Our discussion thread on causality begins with Causality and Circles on May 13. From a Mastodon post by Yohan John, we considered how spatialized diagrams are confused with temporal sequences in a feedback loop. We also covered three papers in this session.
Vernon, D., Lowe, R., Thill, S., and Ziemke, T. (2015). Embodied cognition and circular causality: on the role of constitutive autonomy in the reciprocal coupling of perception and action. Frontiers in Psychology, 6, 1660.
Raginsky, M. (2023). Directed Information and Pearl’s Causal Calculus. arXiv, 1110.0718.
Laland, K.N., John Odling-Smee, J., Hoppitt, W., and Uller, T. (2013). More on how and why: cause and effect in biology revisited. Biological Philosophy, 28, 719–745.
Our conversation continued after the last week of Neuromatch Academy, when the NMA curriculum featured causal networks. Our July 29 meeting featured a collection of references on Bayesianism, Probabilistic Graphical Models, methods of integration, time-series applications, and more. Some core readings are given below.
Stanford Encyclopedia of Philosophy: causal models. This article takes an epistemological approach and provides us with a baseline for structural equation model, graphical probabilistic models, and other statistical formulations of causal relationships.
Daphne Koller’s Probabilistic Graphical Models course. Hosted on Stanford University’s Open Classroom platform, this course includes units on representation, inference, learning, and causation. The causation unit covers decision theory, utility functions, influence diagrams, and the notion of perfect information.
Pearl, J. (2000). Causality. Cambridge Press, Cambridge, UK. This classic book by Judea Pearl builds from a theory of inferred causation, starting at causal diagrams, and continuing through direct effects, indirect effects, confounds, counterfactuals, bounding effects, and probabilities. The book also covers structural models, decision analysis, and Simpson’s Paradox as the basis for methods for detecting causal relationships.
Scholkopf, B. (2019). Causality for Machine Learning. arXiv, 1911.10500.
Heckman, J.J. (2005). The Scientific Model of Causality. Sociological Methodology, 35, 1–97. Causality from an econometrics point-of-view. Counterfactuals are a set of possible outcomes generated by determinants. A causal effect is defined by the change in the manipulated factor where amongst a set of factors, in a situation where all but one is held constant.
Taskesen, E. (2021). A step-by-step guide in detecting causal relationships using Bayesian structure learning in Python. Towards Data Science, September 7.
Which variables have a direct causal effect on a target variable? Hint: association and correlation are not equivalent to causation.
Bayesian Models:
Neuberg, L.G. (2003). Causality: models, reasoning, and inference. Econometric Theory, 19, 675–685.
Pearl, J. (2001). Bayesianism and causality, or, why I am only a half-Bayesian. In “Foundations of Bayesianism”, pgs. 19–36. Kluwer Press.
Methods of Interaction: networks and non-directional graphs, as opposed to directed acyclic graphs (DAGs), require a different set of considerations. The methods below cover highly interacting systems like graphs and how change over time can be properly interpreted as causal.
Leng, S., Ma, H., Kurths, J., Lai, Y-C., Lin, W., Aihara, K., and Chen, L. (2020). Partial cross mapping eliminates indirect causal influences. Nature Communications, 11, 2632.
Park, S.H., Ha, S., and Kim, J.K. (2023). A general model-based causal inference method overcomes the curse of synchrony and indirect effects. Nature Communications, 14, 4287.
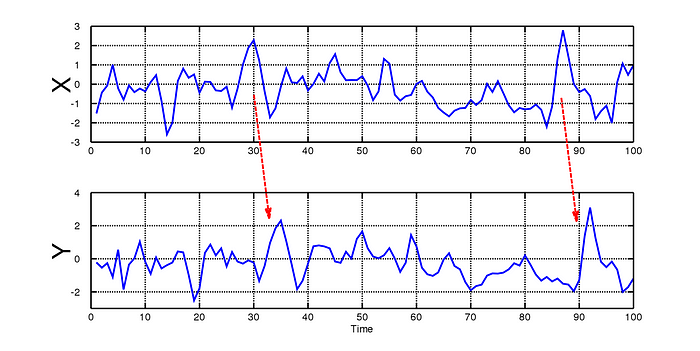
Time-series using Granger Causality: the first two references apply Granger Causality to time-series datasets. In such cases, the datapoints are dependent with respect to time. Given two time-series x and y, x is the cause of y if x predicts y (lagged with respect to x over a certain time interval) given x and prior values of y. This is in comparison with simply predicting the current value of y given previous values of y, which would be the counterfactual case.
The final paper in the group (Stokes and Purdon) critiques Granger Causality from a Neuroscience perspective.
Carlos‐Sandberg, L. and Clack, C.D. (2021). Incorporation of causality structures to complex network analysis of time‐varying behaviour of multivariate time series. Scientifc Reports, 11, 18880.
Runge, J., Nowack, P., Kretschmer, M., Flaxman, S., Sejdinovic, D. (2019). Detecting and quantifying causal associations in large nonlinear time series datasets. Science Advances, 5(11), aau4996.
Stokes, P.A. and Purdon, P.L. (2017). A study of problems encountered in Granger causality analysis from a neuroscience perspective. PNAS, 114(34), E7063-E7072.
The third session (August 5) was a focus on causality specifically as it is treated in Neuroscience. This session followed up on a Twitter debate by Kording Lab and Earl Miller about the role of causality in neuroscience. The consensus to the question “Why is Neuroscience so into causality?” was that it provides a means to identify mechanisms for function. Causality in neuroscience differs from philosophical discussions about causality in that Neuroscience must infer causality from data, while philosophers (and statisticians) do the work of proving causality.
One interesting point from Kording Lab is that there is a difference between proximate causes and ultimate causes. In some fields, causality is obvious and so causal methods are not always necessary. But Neuroscience is partially about the behavioral substrate, and so we can turn to Niko Tinbergen’s four questions. The four questions concern 1) how a trait arose in development (proximate, dynamic), 2) how a trait arose in evolution (ultimate, dynamic), 3) what is the mechanism or structure of a trait (proximate, static), and 4) what is the adaptive value or function of a trait (ultimate, static).
You can read more about Tinbergen’s four questions and their causal implications in the following papers.
Beer, C. (2020). Niko Tinbergen and questions of instinct. Animal Behaviour, 164, 261–265.
Nesse, R.M. (2019). Tinbergen’s four questions: two proximate, two evolutionary. Evolution, Medicine, and Public Health, 2, doi:10.1093/ emph/eoy035.
Mayr, E. (1961). Cause and effect in biology. Science, 134, 1501–1506.
The other papers from this session focused on mental representations and causal functional connectivity in the brain, respectively.
Sloman, S.A. and Lagnado, D. (2015). Causality in Thought. Annual Reviews in Psychology, 66, 223–247.
While Bayesian approaches are good for theory-building, they are an incomplete account of what goes on in the cognitive world.
Biswas, R. and Shlizerman, E. (2022). Statistical perspective on functional and causal neural connectomics: The Time-Aware PC algorithm. PLoS Computational Biology, 18(11), e1010653.
The fourth session (August 19) picks up on a point covered in the second session, namely how causality can be inferred from network data. This covers related ideas of transitivity, weak interactions, and anti-causal models. Papers for this session include networks in ecology, anticipative and non-anticipative control theory, and anti-causal systems.
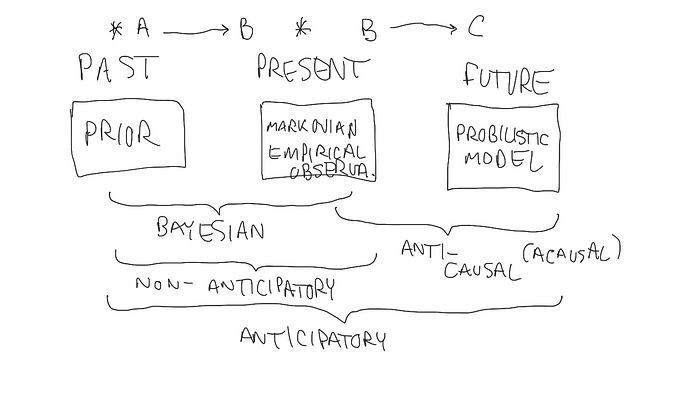
Naghshtabrizi, P. and Hespanha, J.P. (2006). Anticipative and non-anticipative controller design for network control systems. Lecture Notes in Control and Information Science, 331.
Sugihara, G., May, R., Ye, H., Hsieh, C-H., Deyle, E., Fogarty, M., Munch, S. (2012). Detecting Causality in Complex Ecosystems. Science, 338, 496–500.
Chattopadhyay, I. (2014). Causality Networks. arXiv, 1406.6651.
Anticausal System. Wikipedia.
McCurdy, T. (2007). Causal Systems: understanding the basics. Physics Forums. September 23.
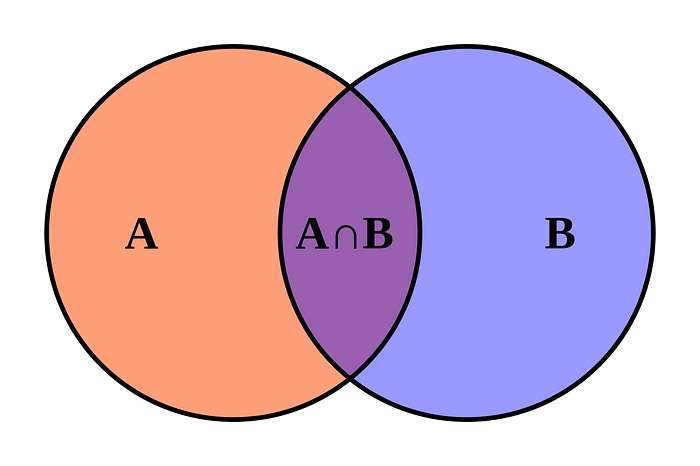
Finally, some fields (cell and molecular biology) have working models of causation that while useful, are not particularly illuminating. In the cell and molecular biology example, the traditional model of necessity and sufficiency (a mechanism being necessary but not sufficient) can be criticized for not being complete with respect to incorporating counterfactuals or multiple potential causes. See this paper for more information:
Bizzarri, M., Brash, D.E., Briscoe, J., Grieneisen, V.A., Stern, C.D., and Levin, M. (2019). A call for a better understanding of causation in cell biology. Nature Reviews Molecular Cell Biology, 20, 261–262.
No comments:
Post a Comment